This transcript has been edited for clarity.
Welcome to Impact Factor, your weekly dose of commentary on a new medical study. I'm Dr F. Perry Wilson of the Yale School of Medicine.
Today I'm going to talk to you about a study at the cutting edge of modern medicine, one that uses an artificial intelligence model to guide care. But before I do, I need to take you back to the late Bronze Age, to a city located on the coast of what is now Turkey.
Troy's towering walls made it seem unassailable, but that would not stop the Achaeans and their fleet of black ships from making landfall, and, after a siege, destroying the city. The destruction of Troy, as told in the Iliad and the Aeneid, was foretold by Cassandra, the daughter of King Priam and Priestess of Troy.
Cassandra had been given the gift of prophecy by the god Apollo in exchange for her favors. But after the gift was bestowed, she rejected the bright god and, in his rage, he added a curse to her blessing: that no one would ever believe her prophecies.
Thus it was that when her brother Paris set off to Sparta to abduct Helen, she warned him that his actions would lead to the downfall of their great city. He, of course, ignored her.
And you know the rest of the story.
Why am I telling you the story of Cassandra of Troy when we're supposed to be talking about AI in medicine? Because AI has a major Cassandra problem.
The recent history of AI, and particularly the subset of AI known as machine learning in medicine, has been characterized by an accuracy arms race.
The electronic health record allows for the collection of volumes of data orders of magnitude greater than what we have ever been able to collect before. And all that data can be crunched by various algorithms to make predictions about, well, anything — whether a patient will be transferred to the intensive care unit, whether a GI bleed will need an intervention, whether someone will die in the next year.
Studies in this area tend to rely on retrospective datasets, and as time has gone on, better algorithms and more data have led to better and better predictions. In some simpler cases, machine-learning models have achieved near-perfect accuracy — Cassandra-level accuracy — as in the reading of chest x-rays for pneumonia, for example.
But as Cassandra teaches us, even perfect prediction is useless if no one believes you, if they don't change their behavior. And this is the central problem of AI in medicine today. Many people are focusing on accuracy of the prediction but have forgotten that high accuracy is just table stakes for an AI model to be useful. It has to not only be accurate, but its use also has to change outcomes for patients. We need to be able to save Troy.
The best way to determine whether an AI model will help patients is to treat a model like we treat a new medication and evaluate it through a randomized trial. That's what researchers, led by Shannon Walker of Vanderbilt, did in a paper appearing in JAMA Network Open.
The model in question was one that predicted venous thromboembolism — blood clots — in hospitalized children. The model took in a variety of data points from the health record: a history of blood clot, history of cancer, presence of a central line, a variety of lab values. And the predictive model was very good — maybe not Cassandra good, but it achieved an AUC of 0.90, which means it had very high accuracy.
But again, accuracy is just table stakes.
The authors deployed the model in the live health record and recorded the results. For half of the kids, that was all that happened; no one actually saw the predictions. For those randomized to the intervention, the hematology team would be notified when the risk for clot was calculated to be greater than 2.5%. The hematology team would then contact the primary team to discuss prophylactic anticoagulation.
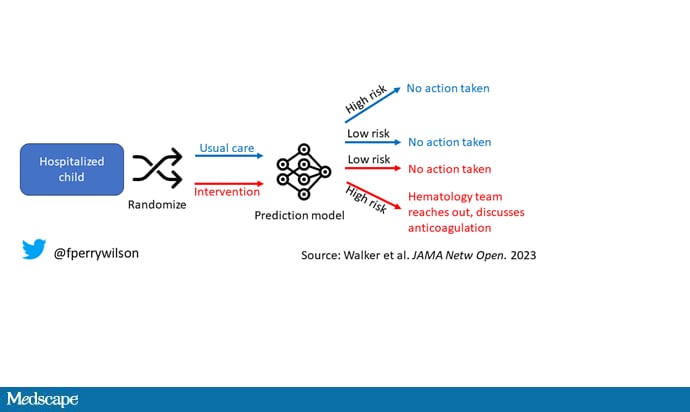
This is an elegant approach. It seeks to answer an important question when it comes to AI models: Does the use of a model, compared with not using the model, improve outcomes?
Let's start with those table stakes — accuracy. The predictions were, by and large, pretty accurate in this trial. Of the 135 kids who developed blood clots, 121 had been flagged by the model in advance. That's about 90%. The model flagged about 10% of kids who didn't get a blood clot as well, but that's not entirely surprising since the threshold for flagging was a 2.5% risk.
Given that the model preidentified almost every kid who would go on to develop a blood clot, it would make sense that kids randomized to the intervention would do better; after all, Cassandra was calling out her warnings.
But those kids didn't do better. The rate of blood clot was no different between the group that used the accurate prediction model and the group that did not.
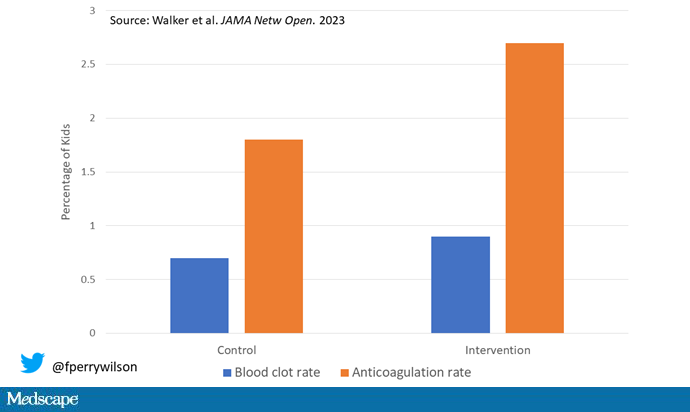
Why? Why does the use of an accurate model not necessarily improve outcomes?
First of all, a warning must lead to some change in management. Indeed, the kids in the intervention group were more likely to receive anticoagulation, but barely so. There were lots of reasons for this: physician preference, imminent discharge, active bleeding, and so on.
But let's take a look at the 77 kids in the intervention arm who developed blood clots, because I think this is an instructive analysis.
Six of them did not meet the 2.5% threshold criteria, a case where the model missed its mark. Again, accuracy is table stakes.
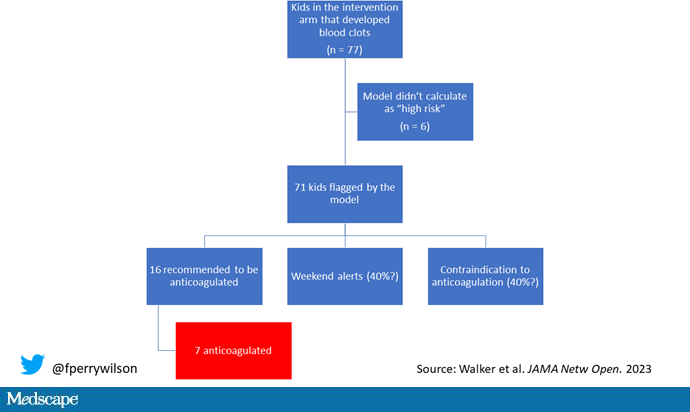
Of the remaining 71, only 16 got a recommendation from the hematologist to start anticoagulation. Why not more? Well, the model identified some of the high-risk kids on the weekend, and it seems that the study team did not contact treatment teams during that time. That may account for about 40% of these cases. The remainder had some contraindication to anticoagulation.
Most tellingly, of the 16 who did get a recommendation to start anticoagulation, the recommendation was followed in only seven patients.
This is the gap between accurate prediction and the ability to change outcomes for patients. A prediction is useless if it is wrong, for sure. But it's also useless if you don't tell anyone about it. It's useless if you tell someone but they can't do anything about it. And it's useless if they could do something about it but choose not to.
That's the gulf that these models need to cross at this point. So, the next time some slick company tells you how accurate their AI model is, ask them if accuracy is really the most important thing. If they say, "Well, yes, of course," then tell them about Cassandra.
F. Perry Wilson, MD, MSCE, is an associate professor of medicine and public health and director of Yale's Clinical and Translational Research Accelerator. His science communication work can be found in the Huffington Post, on NPR, and here on Medscape. He tweets @fperrywilson and his new book, How Medicine Works and When It Doesn't, is available now.
Follow Medscape on Facebook, X (formerly known as Twitter), Instagram, and YouTube
Credits:
Image 1: F. Perry Wilson, MD, MSCE
Image 2: F. Perry Wilson, MD, MSCE
Image 3: F. Perry Wilson, MD, MSCE
Medscape © 2023 WebMD, LLC
Any views expressed above are the author's own and do not necessarily reflect the views of WebMD or Medscape.
Cite this: AI in Medicine Has a Major Cassandra Problem - Medscape - Oct 17, 2023.
Comments